Medical imaging: same quality, less time
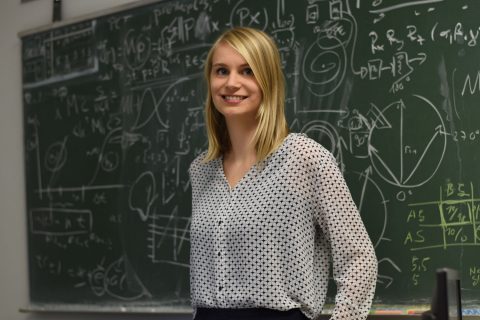
FAU doctoral candidate Elisabeth Hoppe nominated as AI newcomer
Elisabeth Hoppe is completing a doctoral degree at FAU in the area of quantitative MR imaging in combination with deep learning and has developed a deep learning based reconstruction method for the quantitative imaging technique magnetic resonance fingerprinting. In recognition of her research, the scientist from the Pattern Recognition Lab has been shortlisted for the AI newcomer of the year award by the Gesellschaft für Informatik (German Informatics Society). We met to discuss her research approach and what she finds so fascinating about artificial intelligence.
Ms. Hoppe, you have developed a deep learning based reconstruction method for the quantitative imaging technique magnetic resonance fingerprinting (MRF). Can you explain what this means in simple terms?
Magnetic resonance fingerprinting, unlike traditional MR imaging, is a quantitative imaging method. Whilst traditional MR methods deliver images in various shades of grey which only show the differences in contrast in various types of tissue, MRF takes a great many pictures which provide a time series for each pixel. Each pixel is represented by many different time values. The time series varies, showing different patterns depending on the type of tissue which is being investigated. In the case of traditional imaging reconstructions, time series are simulated for the various possible tissue parameters. Every image taken of a patient is then compared with the simulation in order to clarify whether the tissue is healthy or not. The number of comparisons made determines the degree of accuracy. Traditional image reconstruction can take several minutes for each pixel. The great advantage of MRF is that by reconstructing the images we can obtain quantitative information for each position in the image which in turn allows us to evaluate the actual health of the tissue, unlike traditional MR imaging which only shows the different types of tissue.
This is where my research comes in: I want to train neural networks, using deep learning, to reconstruct the images. I am training the networks what tissue looks like at a certain time and in a certain state. This then allows patient images to be evaluated directly without comparing them to the large, simulated basis. This approach is much quicker. It only takes a few milliseconds, but delivers a comparable quality.
How does this technique improve imaging methods, what are the advantages for patients?
We are still at the preliminary stage of our research. Ultimately, however, the reconstructions on the basis of deep learning should be able to be evaluated and discussed directly after the examination, without patients having to wait. Possible areas of application could be brain tumours, epilepsy or prostate cancer.
What do you find so fascinating about artificial intelligence (AI)?
One aspect which I find truly exciting is the great boost in performance compared to traditional methods. There really is no justification for the criticism that the hype about artificial intelligence is exaggerated in this respect. AI techniques in pattern recognition have matured significantly over the last few years. The huge quantities of data generated can easily be processed automatically using AI. It is extremely hard work for doctors to inspect all individually segmented images and differentiate between healthy and non-healthy tissue. They become tired, and may make mistakes. Artificial intelligence delivers just as good results as a doctor, and never tires. This is what drives me and motivates me to use AI in other areas such as image reconstruction.
It’s also fascinating to see how the world reacts to AI: it will have a profound effect not only on industry and science, but also on society as a whole. AI is being used in more and more areas: image reconstruction, segmentation, supporting doctors in making a diagnosis, and there are so many more possibilities which have not yet been researched.
What makes FAU such a significant location for AI research, in particular in the field of medical engineering?
Above all the very good connections between FAU and the surrounding industry, especially Siemens, which is also extremely important for my work. This provides us with significant input which we can integrate into our research with a view to future applications. Universitätsklinikum Erlangen also has an important role to play. It is a great source of innovative input for us as researchers. International collaboration is also extremely valuable, for example between the Pattern Recognition Lab, where I am based, and John Hopkins University in the USA.
The fact that FAU offers the entire spectrum of academic disciplines is another significant aspect. Doctoral candidates and researchers from other institutes are just a stone’s throw away, it is easy to meet and get a new spin on research projects or provide support for one another. It is an inspirational research environment.
Voting for AI newcomer of the year
The project ‘#KI50: Künstliche Intelligenz in Deutschland – gestern, heute, morgen’ (#AI50: Artificial Intelligence in Germany – yesterday, today, tomorrow’), run by Gesellschaft für Informatik e.V. (German Informatics Society), is turning the spotlight on young, committed AI researchers from all disciplines. Ten outstanding AI newcomers will then be recognised within the context of the Federal Ministry of Education and Research’s ‘Science Year 2019 – Artificial Intelligence’. Votes can be cast until 17 November. Further information is available on the website.
You can vote directly for Elisabeth Hoppe here.
Further information:
Elisabeth Hoppe
Phone: + 49 9131 8527874
elisabeth.hoppe@fau.de