Recognizing arthritis in an MRI scan
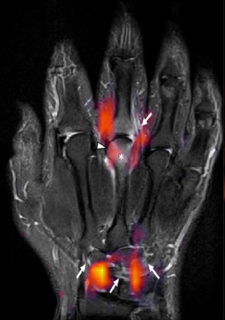
FAU team of researchers use artificial intelligence to analyze MRI scans and classify various types of arthritis.
Arthritis is a widespread condition affecting hundreds of thousands of people that leads to inflammation of the joints. It has many different causes, and if physicians are to treat the disease properly, it is important that they can determine exactly which type of arthritis the patient has. This is often no easy undertaking. A number of different parameters have to be considered and a definite diagnosis is often only possible as the disease progresses.
Computer scientists from the Chair of Computer Science 5 Pattern Recognition at FAU and physicians from Department of Medicine 3 – Rheumatology and Immunology and the Institute of Radiology at Universitätsklinikum Erlangen have conducted a study to investigate whether neural networks can determine whether a patient is suffering from rheumatoid arthritis (RA) or psoriatic arthritis (PsA). The outcome: AI was able to differentiate between the two types in 75 percent of cases.
The team had only recently investigated whether neural networks could determine the type of arthritis using high resolution computer tomography images. They were successful. According to Prof. Frank Roemer from the Institute of Radiology, “the advantage of MRI compared to CT is that an MRI scan gives a more accurate picture of the extent of the inflammation and the affected joint structures.”
For the study, the team led by computer scientist Lukas Folle used five different MRI sequences from 649 patients to train and test an innovative neural network. The network was able to classify the type of arthritis patients were suffering from in 75 percent of the cases based on the MRI images.
Furthermore, the team tested how the neural network classified cases of psoriasis, which can often progress into PsA. The network correctly classified most of the psoriasis cases that later developed into PsA as PsA.
Folle believes “it is possible that the neural network picks up on early changes or other structural features in psoriasis patients and uses these to classify them accordingly.
Our results indicate that MRI scans can show changes that the neural network has identified as being relevant for classifying the various forms of arthritis and that have not yet been described to date,” adds PD. Dr. David Simon, a physician involved in the study. “We now aim to continue training and improving the neural network, with an view to it possibly being used in clinical practice,” explains Lukas Folle.
The team has now published their results in a leading journal for rheumatology and immunology (Rheumatology).
Further information
Lukas Folle
Chair of Computer Science 5 (Pattern Recognition)
Phone: +49 9131 85 28982
lukas.folle@fau.de
Prof. Frank Roemer
Institute of Radiology
frank.roemer@uk-erlangen.de
PD Dr. Arnd Kleyer
Chair of Internal Medicine III
Phone: +49 9131 85 32093
arnd.kleyer@uk-erlangen.de
PD Dr. David Simon
Chair of Internal Medicine III
Phone: +49 9131 85 32093
david.simon@uk-erlangen.de